As industries continue to embrace digital transformation, RUL has emerged as a game-changer in optimizing resource allocation and extending the lifespan of critical assets. By leveraging real-time data and advanced algorithms, companies can now predict failures before they occur, minimizing disruptions and ensuring seamless operations. This guide will delve deep into the concept of RUL, exploring its applications, methodologies, and future trends while providing actionable insights for businesses and professionals alike. Whether you're a seasoned engineer, a data scientist, or a business leader looking to implement predictive maintenance strategies, this comprehensive article will serve as your go-to resource for mastering RUL. From understanding the underlying principles to exploring practical use cases, we'll cover everything you need to know to harness the power of RUL effectively. So, let’s dive in and explore how RUL can revolutionize your approach to asset management and operational efficiency.
Table of Contents
- What is RUL and Why Does It Matter?
- How is RUL Calculated? A Step-by-Step Guide
- Applications of RUL Across Industries
- What Tools and Technologies Are Used for RUL Prediction?
- What Are the Challenges in Implementing RUL?
- Future Trends in RUL and Predictive Maintenance
- Best Practices for Maximizing RUL Benefits
- Frequently Asked Questions About RUL
What is RUL and Why Does It Matter?
Remaining Useful Life (RUL) is a metric that quantifies the time remaining before a system or component is expected to fail. It is a cornerstone of predictive maintenance, enabling organizations to anticipate failures and take corrective actions before they occur. RUL is particularly valuable in industries where equipment downtime can result in significant financial losses or safety risks, such as aviation, manufacturing, and healthcare. The importance of RUL lies in its ability to transform maintenance strategies from reactive to proactive. Traditionally, maintenance schedules were based on fixed intervals or occurred only after a failure had happened. However, with RUL, organizations can optimize maintenance activities, reducing unnecessary inspections while ensuring timely interventions. This not only extends the lifespan of assets but also improves operational efficiency and reduces costs.
Why Should Businesses Care About RUL?
Businesses that adopt RUL-driven strategies gain a competitive edge by minimizing downtime and maximizing asset utilization. For instance, in the aviation industry, predicting the RUL of aircraft engines allows airlines to schedule maintenance during off-peak hours, avoiding costly delays. Similarly, in manufacturing, RUL helps prevent unplanned shutdowns, ensuring uninterrupted production. By integrating RUL into their operations, businesses can achieve higher reliability, enhanced safety, and improved profitability.
Read also:How Much Is Damon Wayans Net Worth In 2023 A Comprehensive Overview
How is RUL Calculated? A Step-by-Step Guide
Calculating RUL involves a combination of data collection, analysis, and modeling. The process typically begins with gathering data from sensors, historical records, and other sources. This data is then processed using advanced algorithms to identify patterns and predict future behavior. Below is a step-by-step guide to understanding how RUL is calculated:
Step 1: Data Collection
The first step in calculating RUL is collecting relevant data. This includes:
- Sensor data: Information from IoT devices monitoring temperature, vibration, pressure, and other parameters.
- Historical data: Past performance and failure records of similar assets.
- Environmental data: External factors like weather conditions or operational stress that may impact asset performance.
Step 2: Data Preprocessing
Once the data is collected, it needs to be cleaned and preprocessed. This involves:
- Removing outliers and noise from sensor readings.
- Normalizing data to ensure consistency across different sources.
- Segmenting data into meaningful time intervals for analysis.
Why Is Data Preprocessing Crucial for Accurate RUL Prediction?
Data preprocessing ensures that the input to predictive models is accurate and reliable. Without proper preprocessing, anomalies or inconsistencies in the data can lead to incorrect RUL estimates, resulting in suboptimal maintenance decisions. For example, a sudden spike in temperature readings due to a sensor malfunction could falsely indicate an impending failure if not addressed during preprocessing.
Step 3: Model Selection and Training
The next step is selecting an appropriate model for RUL prediction. Common approaches include:
- Machine learning models: Algorithms like Random Forest, Support Vector Machines, and Neural Networks are widely used for RUL prediction.
- Physics-based models: These rely on understanding the physical processes governing asset degradation.
- Hybrid models: Combining machine learning and physics-based approaches for enhanced accuracy.
Applications of RUL Across Industries
RUL has found applications in a wide range of industries, each leveraging its predictive capabilities to improve efficiency and reliability. Below are some notable examples:
Read also:Discover The Beauty Of Wasmo Somali A Cultural And Artistic Treasure
Aviation
In the aviation sector, RUL is used to predict the remaining lifespan of critical components like engines, landing gear, and avionics. By accurately estimating RUL, airlines can optimize maintenance schedules, reduce downtime, and enhance passenger safety.
Manufacturing
Manufacturers use RUL to monitor the health of machinery and production lines. Predicting RUL allows them to schedule maintenance during planned downtimes, avoiding costly unplanned stoppages and ensuring consistent output.
Energy
In the energy sector, RUL is applied to wind turbines, power plants, and other infrastructure. By predicting failures in advance, energy companies can minimize disruptions and ensure a stable supply of electricity.
What Tools and Technologies Are Used for RUL Prediction?
Predicting RUL requires a combination of advanced tools and technologies. These include software platforms, hardware devices, and analytical frameworks designed to process and interpret complex data sets.
Software Platforms
Several software platforms are available for RUL prediction, including:
- IBM Maximo: A comprehensive asset management solution that integrates RUL prediction with maintenance planning.
- Siemens MindSphere: An IoT platform that leverages machine learning for predictive maintenance and RUL estimation.
- Microsoft Azure IoT: A cloud-based platform offering tools for data analytics and RUL modeling.
Hardware Devices
Hardware devices such as sensors and IoT gateways play a crucial role in collecting real-time data for RUL prediction. These devices are often equipped with advanced features like edge computing to process data locally before transmitting it to centralized systems.
How Do These Tools Work Together to Enhance RUL Accuracy?
The integration of software and hardware tools creates a seamless ecosystem for RUL prediction. Sensors collect data, which is then processed by IoT gateways and transmitted to cloud platforms for analysis. Machine learning algorithms analyze this data to generate accurate RUL estimates, enabling timely maintenance interventions.
What Are the Challenges in Implementing RUL?
While RUL offers numerous benefits, its implementation is not without challenges. These challenges can hinder the accuracy and effectiveness of RUL predictions, requiring careful planning and execution.
Data Quality Issues
One of the primary challenges in RUL implementation is ensuring data quality. Poor-quality data, such as incomplete or inconsistent readings, can lead to inaccurate predictions. Addressing this requires robust data collection and preprocessing strategies.
Model Complexity
RUL models, especially those based on machine learning, can be complex and resource-intensive. Developing and training these models requires specialized expertise and computational power, which may not be readily available in all organizations.
Integration with Existing Systems
Integrating RUL prediction tools with existing maintenance and asset management systems can be challenging. Organizations must ensure compatibility and seamless data flow between different platforms to maximize the benefits of RUL.
Future Trends in RUL and Predictive Maintenance
The field of RUL and predictive maintenance is rapidly evolving, driven by advancements in technology and increasing demand for efficiency. Below are some key trends shaping the future of RUL:
AI and Machine Learning Advancements
AI and machine learning are expected to play an even greater role in RUL prediction. Enhanced algorithms and increased computational power will enable more accurate and real-time predictions, further optimizing maintenance strategies.
Edge Computing
Edge computing is gaining traction as a way to process data closer to the source, reducing latency and improving RUL accuracy. By analyzing data locally, organizations can make faster and more informed decisions.
Digital Twins
Digital twins, virtual replicas of physical assets, are becoming increasingly popular for RUL prediction. These models simulate real-world conditions, providing deeper insights into asset performance and degradation.
Best Practices for Maximizing RUL Benefits
To fully harness the potential of RUL, organizations should adopt best practices that ensure accurate predictions and effective implementation. These include:
Investing in Data Quality
High-quality data is the foundation of accurate RUL predictions. Organizations should prioritize data collection and preprocessing to eliminate errors and inconsistencies.
Continuous Model Improvement
RUL models should be continuously updated and refined based on new data and insights. This ensures that predictions remain accurate and relevant over time.
Training and Education
Providing training and education to employees on RUL concepts and tools is essential for successful implementation. This empowers teams to leverage RUL effectively in their operations.
Frequently Asked Questions About RUL
What is the Difference Between RUL and Predictive Maintenance?
While RUL is a specific metric used to estimate the remaining lifespan of an asset, predictive maintenance is a broader strategy that uses RUL and other metrics to optimize maintenance activities.
How Can Small Businesses Benefit from RUL?
Small businesses can use RUL to extend the lifespan of their equipment, reduce maintenance costs, and improve operational efficiency, even with limited resources.
Is RUL Applicable to Non-Technical Industries?
Yes, RUL can be applied to non-technical industries like retail and healthcare by predicting the lifespan of assets like HVAC systems or medical equipment, ensuring timely replacements and minimizing disruptions.
Conclusion
In conclusion, Remaining Useful Life (RUL) is a transformative concept that empowers organizations to predict failures, optimize maintenance, and enhance operational efficiency. By leveraging advanced tools and technologies, businesses across industries can unlock the full potential of RUL, driving innovation and growth. As the field continues to evolve, staying informed about the latest trends and best practices will be key to maximizing RUL benefits.
For further reading on predictive maintenance and RUL, you can explore resources like IBM Maximo, a leading platform for asset management and RUL prediction.
By adopting RUL-driven strategies, organizations can not only reduce costs but also build a culture of proactive decision-making, ensuring long-term success in an increasingly competitive landscape.
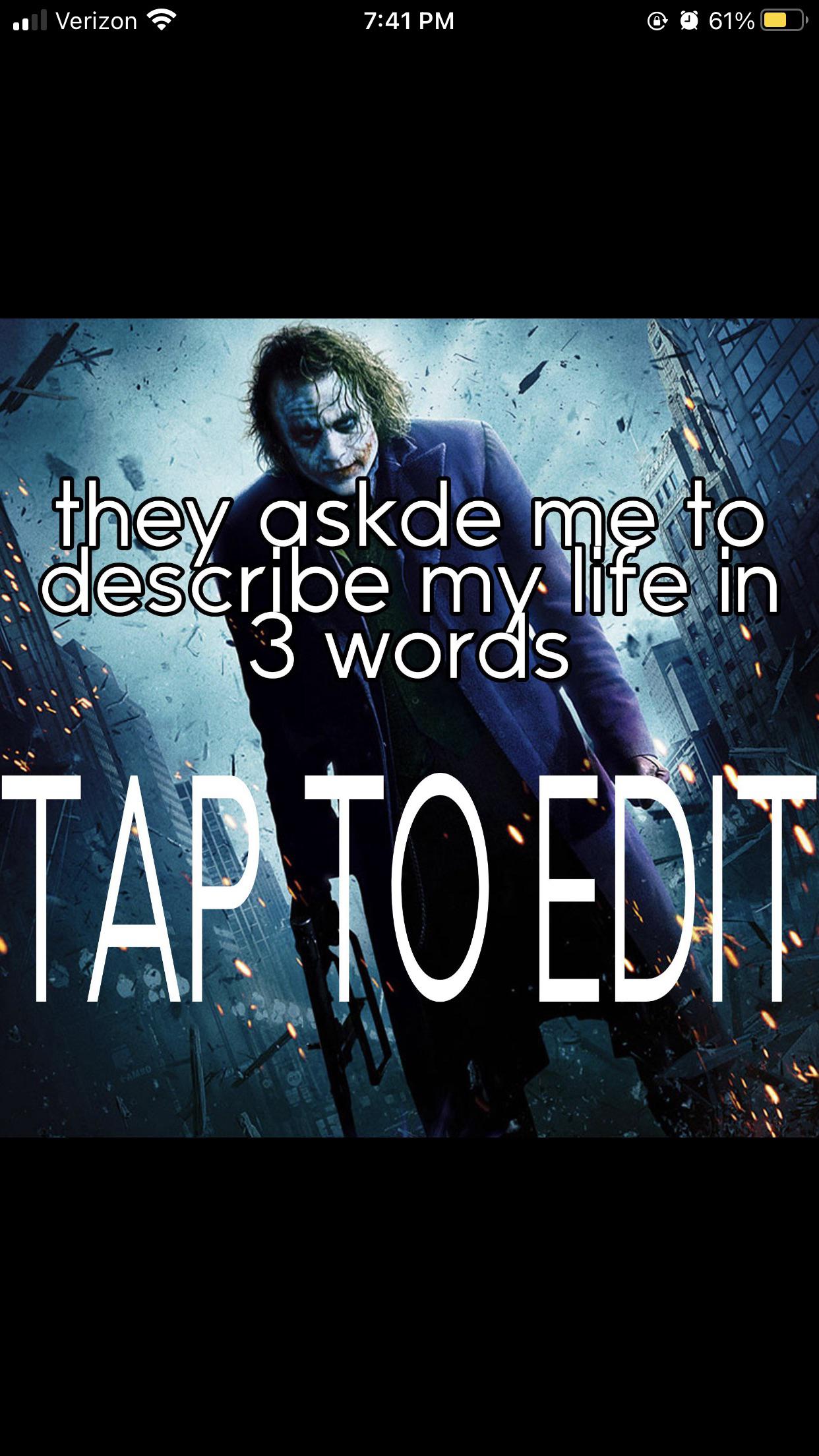
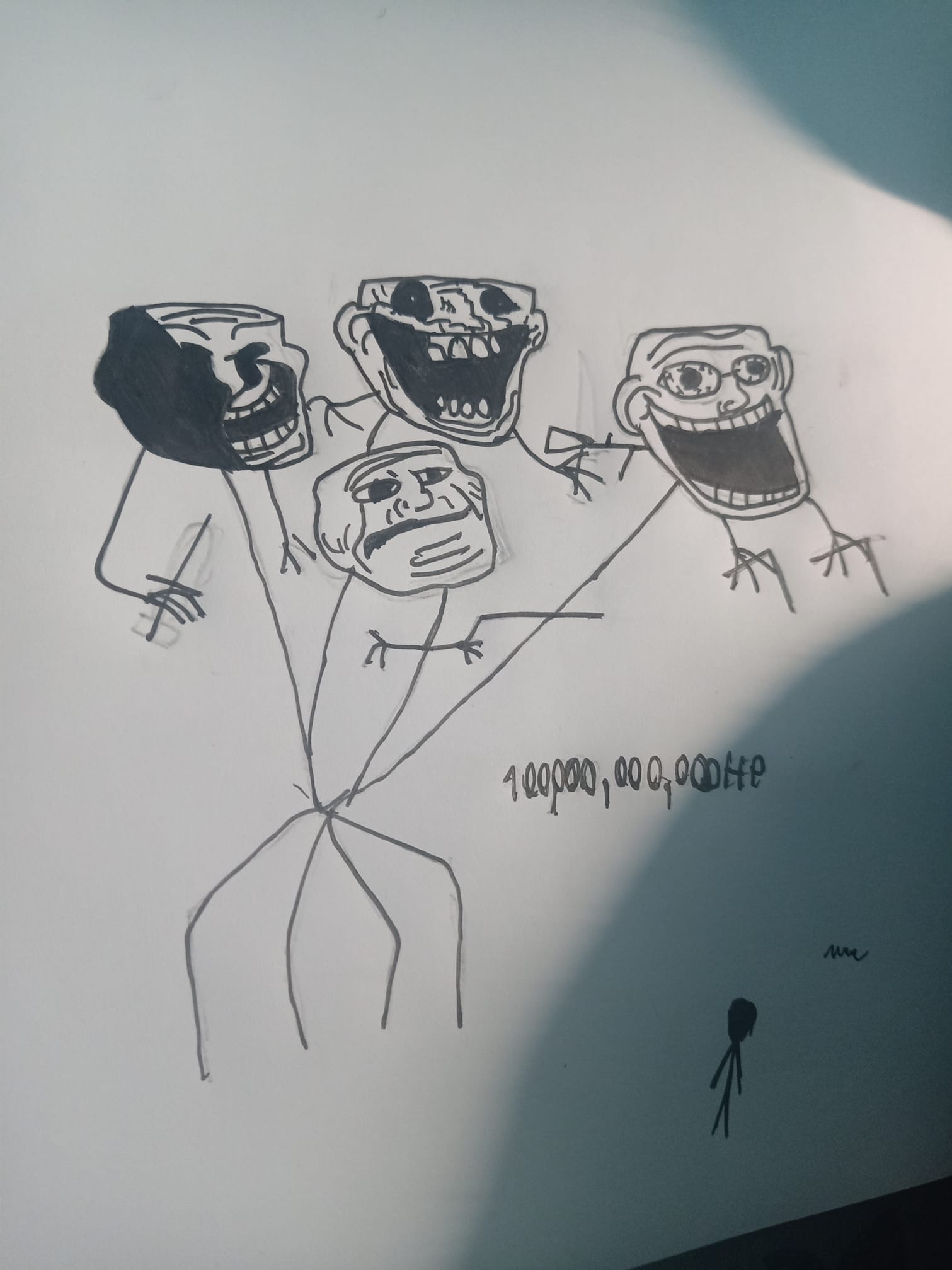